Stop chasing AI benchmarks—create your own
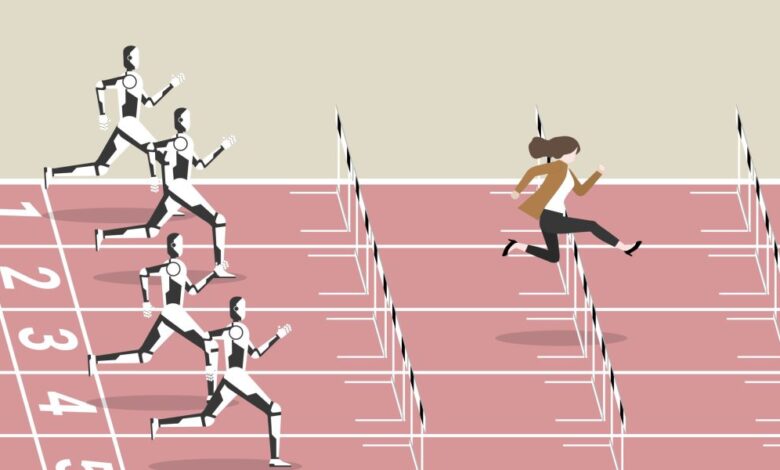

Each a few months, the new large language model (LLM) was cleared of the hero of artificial intelligence, with standard degrees. But these famous standards for LLM performance-such as a thinking test at the level of graduate studies and abstract mathematics-reflects except for real work needs or really represent new AI borders. For companies on the market for the Models of Amnesty International for Institutions, based on the decision of the models that must be used on these top panels alone can lead to costly errors-from lost budgets to unprecedented capabilities and potential harmful errors that are rarely acquired by standard degrees.
General standards can be useful for individual users by providing directional indicators of artificial intelligence capabilities. It is recognized that some criteria for completing the programming instructions and program engineering, such as Swe-Bencer or Codeforcees, are valuable to companies within a narrow range of coding applications. But the most common criteria and public top panels often spend the attention of companies and developers for models, pushing innovation towards marginal improvements in unhelpful areas of companies or have nothing to do with the fields of innovation of artificial intelligence.
Therefore, the challenge of executives lies in designing the evaluation frameworks for businesses that test the possible models in the environments that will be already published. To do this, companies will need to adopt assessment strategies designed to operate them widely using relevant and realistic data.
Lack of compatibility between standards and work needs
The delightful criteria described by models developers in their releases are often separated from the facts of institutions applications. Consider some of the most popular: thinking at the graduate level (GPQA Diamond) and mathematics tests at high school, such as Math-500 and AIME2024. Each of these was martyred in publications GPT O1and Sonnet 3.7Or Deepseek’s R1. But none of these indicators is useful in evaluating the applications of joint institutions such as knowledge management tools, design assistants, or Chatbots facing the customer.
Instead of assuming that the “best” model on the designated leaders plate is the clear option, companies must use the measures specifically designed for their own needs to work back and determine the correct model. Start by testing models on the context and actual data-real customer information, the field documents, or any inputs your system will face your production. When real data is rare or sensitive, companies canCraft artificial testing casesThat picks up the same challenges.
Without real world tests, companies can end up with inappropriate models, for example, a lot of memory requires Edge devices, or have a very high transition time for actual time reactions, or you do not have enough support for publication while discrimination sometimes according to data governance standards.
Salesforce tried to fill this gap Between joint standards and their actual business requirements through Growth Its internal standard for its CRM needs. The company has established its special assessment criteria for tasks such as excavation, expected customer care, and generating service summaries – the actual work that marketing and sales teams need for Amnesty International.
Reaching beyond the standards are flowing
common The criteria are not only enough to make the enlightened decisions of the business, but it can be misleading as well. Often LLM media coverage, including everyone three major Modern version ads, standards are used to compare models based on middle performance. The specified criteria are distilled to a One point, number or tape.
The problem is that the models of the intrusive intelligence are random and sensitive systems, which means that the slight differences of a claim can make them act unexpectedly. accident Antarbur search paper He truly argues that, as a result, the individual points on the performance comparison scheme are insufficient due to the large error ranges of evaluation standards. A recent study conducted I found microsoft The use of the assessment based on the most statistically accurate groups in the same criteria can significantly change the ranking of the ranking of the general accounts on the of the leaders of the leaders.
For this reason, business leaders need to ensure reliable measurements to perform the model via a reasonable set of differences, which are widely done, even if they require hundreds of test operations. This accuracy becomes more important when multiple systems are combined throughData supply chains and dataIt is possible to increase the contrast. For industries such as aviation or health care, the error margin is small and exceeds the current criteria that are included in the current standards, so that dependence on the top scales can depend only great operational risks in publishing operations in the real world.
Companies must also test models in litigation scenarios to ensure security and a model – such as Chatbot resistance to manipulation by bad actors trying to bypass handrails – that cannot be measured by traditional standards. Llms is Significantly exhibition To deceive it with advanced claim techniques. Depending on the state of use, the implementation of strong guarantees against these weaknesses can determine the technology selection strategy and publishing strategy. The elasticity of a model in the face of a possible bad actor can be a more important measure of mathematics capabilities or thinking about the model. From our point of view, artificial intelligence “guaranteed” is an exciting and effective obstacle to breaking artificial intelligence researchers, which may require techniques for developing and testing a new model.
Evidence mode: Four keys for a developed approach
Start with the current evaluation frameworks. Companies must start taking advantage of the strengths of the current automated tools (along with human rule and practical but repetitive measurement objectives). Specialized evaluation tools for evaluation tools, such as deepand Langsmithand trutlensand MasteryOr ArtkitThe test can be accelerated, simplified, and allowed a fixed comparison through models and over time.
Bring human experts to the test. Effective artificial intelligence evaluation requires that the automated test be completed with human rule whenever possible. Automated evaluation can include a comparison between LLM answers to the atmosphere of earth Marry or Blue Grades, to measure the quality of the text summary.
For accurate assessments, however, those that are still struggling machines, human evaluation is still vital. This can include the experts of the two fields or the final users who review a “blind” sample of the model’s outputs. Such procedures can also indicate possible biases in responses, such as LLMS giving responses to biased jobs by sex or race. This human layer of heavy labor review, but it can provide an additional critical vision, such as whether the response is already beneficial and a good institution.
The value of this hybrid approach can be seen in a Modern case study Where the Chatbot company evaluated human resources support using both human and mechanical tests. The company’s internal evaluation of the company with human participation showed an important source of LLM’s response errors due to the defective updates of the institution’s data. The discovery sheds light on how to reveal the human evaluation of the regular issues that go beyond the same model.
Focus on the bodies, not the dimensions of isolated evaluation. When evaluating the forms, companies should consider beyond precision to consider the full spectrum of work requirements: speed, cost efficiency, operational feasibility, flexibility, maintenance, and organizational compliance. The model, which is better marginalized, may be very expensive or very slow accuracy standards for applications in actual time. A great example is how GPT O1 opens from artificial intelligence (a leader in many criteria at the time of release) It is implemented when applied to the ARC-Eagi Award. To surprise many, the O1 model performs badly, due to the “ARC-AGI” on the computing power used to solve standard tasks. Often the O1 O1 takes a long time, using more time to calculate to try to reach a more accurate answer. The most popular standards do not have a time limit although the time will be an important factor for many business use.
Blinds become more important in the growing world of applications (multiple), as the most simplicity of tasks can be dealt with with cheaper and faster models (supervised by a coincidence agent), while the most complex steps (such as solving a series of absolute problems of the customer) can need a more powerful version with successful thinking.
Microsoft ResearchHugingGPTFor example, it organizes specialized models for different tasks under the central language model. Preparing to change models for different tasks requires elaborate tools that do not symbolize one model or one provider. This integrated flexibility allows the axis of the models and change them easily based on the evaluation results. Although this may seem to be a lot of additional development work, there are a number of tools available, such asLinjshenandLlamaindexAndYour pydanticThe process can be simplified.
Turn the model test to the culture of continuous evaluation and monitoring. With the development of technology, continuous evaluation ensures that artificial intelligence solutions remain ideal while maintaining compatibility with work objectives. It is very similar to how to implement software engineering teams for continuous integration and slope test to arrest errors and prevent the deterioration of performance in the traditional code. Artificial intelligence systems require a regular evaluation against business standards. Similar to the pharmaceutical activation between users of new drugs, you should also collect and analyze reactions from LLM users and stakeholders affected continuously to ensure “behavior” as expected “and Do not get out of the intended performance goals.
This type of detailed assessment frame enhances the culture of experimentation and data -based decisions. It also imposes a new and decisive talisman: artificial intelligence can be used for implementation, but humans are in control and artificial intelligence must be governed.
conclusion
For business leaders, the path does not lie to the success of artificial intelligence in chasing the latest measurement heroes, but in developing evaluation frameworks for your specified business goals. Think about this approach as “the leaders of each user”, as oneStanford paper suggests.The true value of spreading artificial intelligence comes from three main procedures: determining the measures that measure success directly in the context of your work; Implement the statistically strong test in realistic situations using your actual data and in your actual context; And promoting the culture of monitoring, evaluation and continuous experimentation that depends on automated tools and human experience to assess the bites through models.
By following this approach, executive managers will be able to determine improved solutions to meet their own needs without paying outstanding prices for “first -class models”. We hope to do this in directing the models development industry away from chasing marginal improvements on the same standards – the victim is broken by Goodhart Law with limited use of businesses – instead editing them to explore new ways of innovation and launch the following artificial intelligence.
Read last luck Pillars for Franlone Franlon.
Francois Candelon A partner at SEVEN2 Special Stock Company and former World Director of the BCG Henderson Institute.
Theodorus Evgeniou He is a professor at Insead and founder of Trust and Safety Tremau.
Max Struever is a major engineer in BCG-x A ambassador at the BCG Henderson Institute.
David Zuluhaga Martinez, partner in Boston Consulting Group A ambassador at the BCG Henderson Institute.
Some of the companies mentioned in this column are former or current customers for the authors’ work.
This story was originally shown on Fortune.com
https://fortune.com/img-assets/wp-content/uploads/2025/04/GettyImages-1453524742-e1743632840375.jpg?resize=1200,600
2025-04-04 09:30:00