Anthropic makes a breakthrough in opening AI’s ‘black box’
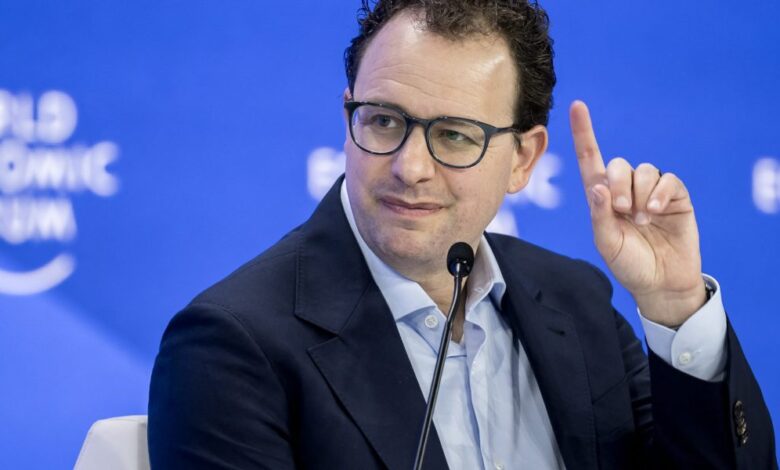
Researchers at the Artificial Intelligence Company man Suppose they have achieved a fundamental breakthrough in our understanding of large language models, the type of artificial intelligence responsible for the current boom, and work. This penetration has important effects on how we can make artificial intelligence models safer, safer and more reliable in the future.
One of the problem is the strong artificial intelligence today, which depends on the large language models (LLMS) that the models are black boxes. We can know what is demanding their feeding and the outputs they produce, but exactly how they reach any specific response is a mystery, even for artificial intelligence researchers who build them.
This ambiguity creates all kinds of issues. It makes it difficult to predict when it is likely to “models of hallucinations”, or broadcast with wrong information. We know that these large artificial intelligence models are vulnerable to various prison scraps, as they can be deceived in jumping on handrails (the developers of the artificial intelligence model limits to revolve around the outputs of the model so that he does not use the racist language or write a malicious person or tell them how to build a bomb). But we do not understand the reason why some prisons work better than others, or why it does not result from the control that is used to create strong inhibitors enough to prevent models whose developers do not want to do so.
Our inability to understand how LLMS works for some companies It is hesitant to use it. If the interior of the models is more understanding, companies may give more confidence to use models on a wider scale.
There are effects on our ability to keep controlling the increasing “agents” of artificial intelligence. We know that these agents are able to “reward the piracy” – which makes ways to achieve a goal that was not the user of the intended model. In some cases, models can be deceptive, lying to users about what they did or trying to do. Although the latest “logical” artificial intelligence models produce what is known as “a series of thought”-a kind of plan for how to answer a claim that includes what is looking for a person such as “self-thinking”-we do not know whether the series of thought represented by the model is precisely the steps it takes (and there is often evidence that it may not be so.)
The new search for Anthropic provides a way to solve some of these problems at least. Create A new tool for decoding how “Think” LLM. In essence, what the anthropron researchers built somewhat similar to the nerve tests that neuroscientists use to survey the brains of human research topics and detect the brain areas that seem to play a larger role in various aspects of perception. After this tool that resembles functional magnetic resonance was invented, anthropology applied it to the Claude 3.5 haiku model of man. When doing this, they managed to solve many major questions about how Claude works, and perhaps most other LLMS.
The researchers found Although although LLMS like Claud is initially trained to predict the following word in the sentence, Claud in this process learns to do some long -term planning, at least when it comes to certain types of tasks. For example, when she is asked to write a poem, Claude finds logical words with the subject of the poem or its subject that he wants to jump and then works back to create a sentence that will end with these rhyme words.
They also found that Claude, who was trained to be multi -language, has no completely separate ingredients to think about every language. Instead, common concepts are included across languages in the same collection of neurons within the model and the model appears to be a “cause” in this conceptual space, then only convert the director into the appropriate language.
The researchers also discovered that Claude is able to lie about his series of ideas to satisfy the user. The researchers showed this by asking the model about a problem with difficult mathematics, but then giving the model an incorrect hint about how it is solved.
In other cases, when asking a question is easier that the model can answer more or less immediately, without the need for reason, the model constitutes a fake thinking process. “Although she claims to manage an account, our interpretation techniques do not reveal any evidence in all this after its occurrence,” Josh Baston, Anthropor researcher worked on the project.
The ability to track the internal thinking of LLMS opens new possibilities for auditing artificial intelligence systems for safety and safety fears. Researchers may also help develop new training methods to improve handrails owned by artificial intelligence systems and reducing hallucinations and other defective outputs.
Some artificial intelligence experts refuse the “black box problem” in LLM by saying that human minds are also mysterious with other humans, yet we rely on humans throughout the day. We cannot really know what another person thinks-in reality, psychologists have shown that sometimes we do not understand how our own thinking works, which shows logical interpretations after reality to justify the procedures that we provide either intuitively or to a large extent due to the emotional responses that we may not be aware of. We often mistakenly assume that another person thinks more or less in the same way we do – and that can lead to all kinds of misunderstanding. But it also seems that, in the words of, on a large scale, humans tend to think in somewhat similar ways, and that when we make errors, these errors fall into somewhat familiar patterns. (This is why psychologists have been able to determine many common cognitive biases. However, the problem in LLMS is that the way you reach outputs seem strange enough for how humans perform the same tasks that they can fail in ways that are unlikely to be for a person.
Paston said that thanks to the types of techniques he and other scientists develop to investigate these exotic LLM brains – a field known as “mechanical interpretation” – Rapid’s progress is made. He said: “I think in another year or two, we will know more about how these models think more than we think about how people think.” “Because we can only do all the experiences we want.”
Previous techniques to try to investigate how LLM works Focus either trying to decode individual neurons or small groups of neurons within the neuroma, or order layers of the neuron that sits under the final output layer to rid the resulting output, and reveal something about how to treat the model. Other methods of “eradication” – mainly remove the nerve network cut – then compare how the model performs with how it originally performed.
What Antarbur did in his new research is actually training a completely different model, called CLT layer coding, which works using groups of interpretable features instead of individual neurons weight. An example of these features may be all associated with a specific action, or any term indicating “more than”. This allows researchers a better understanding of how the model is working by allowing them to determine complete “circles” of neurons that tend to tie together.
“Our model is analyzed, so we get new pieces, not similar to the original neurons, but there is pieces, which means that we can actually see how different parts play different roles,” said Baston. “It also has the advantage of allowing researchers to track the entire thinking process through the network layers.”
However, a person said that the method has some defects. It is just an approximation of what is already happening within a complex model like Claude. There may be neurons located outside the circles, the CLT method that plays some accurate but decisive sessions in formulating some of the model’s outputs. The CLT technology also does not pick up a major part of how LLMS works – a thing that is called attention, as the model learns to put a different degree of importance on different parts of the input router during the formulation of its output. This attention turns dynamically as the form formulates its output. CLT cannot capture these transformations in attention, which may play an important role in “thinking”.
Anthropor also said that the discrimination of the network circles, even for demands that are only “dozens of words”, take a human expert for several hours. He said it is not clear how the technique can be reduced to treat claims that were much longer.
This story was originally shown on Fortune.com
https://fortune.com/img-assets/wp-content/uploads/2025/03/GettyImages-2194801091-e1743089710921.jpg?resize=1200,600
2025-03-27 17:00:00